Ivo Velitchkov (2023) Personal Knowledge Graphs #개인지식그래프
2024-07-20 Bibliography bib knowledgegraph pkg c006Personal Knowledge Graphs : Connected thinking to boost productivity, creativity and discovery
(Ivo Velitchkov and George Anadiotis 2023)
- “Personal Knowledge Graphs: Connected thinking to boost productivity, creativity and discovery” Ivo Velitchkov and George Anadiotis 2023
Is your thinking connected?Do you write, read, research and think for work or leisure? Then you’ll have years of notes, ideas, articles and images. But all those thoughts are decaying. They are stuck in dusty notebooks, forgotten files on old backups and buried emails.What if…all the thinking you had ever done was live, fresh and connected?adding new knowledge popped up connections to writing and reading you had forgotten?you could travel through your thoughts like surfing the web?That is connected thinking. That is a Personal Knowledge Graph.In Personal Knowledge Graphs, experts and researchers explore the latest uses of PKGs. We mine the bumps to productivity, creativity and serendipity that come from a PKG practice. And delve into new developments and novel ways of thinking about and using PKGs to go beyond just linking topics and text.Want to expand your mind and go deeper with PKGs?Personal Knowledge Graphs: Connecting Thinking to Boost Productivity, Creativity and Insight will link you to the cutting edge of tools for thought.Praise for Personal Knowledge Graphs: Connected thinking to boost productivity, creativity and discoveryAs a productivity coach, I use PKGs primarily for making knowledge actionable—which has implications for the intake, development, and output of knowledge. The essays Velitchkov and Anadiotis have assembled in Personal Knowledge Graphs cover a wide variety of important PKG topics. Some essays are more philosophical, some are more pragmatic, but all of them deepened my understanding of how I can get the most out of the PKG tools I use.— R.J. Nestor, Productivity in Tools for Thought expert——————Knowledge Graphs are now widely accepted in industry and government as an effective way to combine, store and query large volumes of heterogeneous data. This book is the first to open the door to a new application of knowledge graphs: individual citizens that want to have control over their own data, with applications ranging from personal archiving all the way up to a personal digital assistant. The book is a collection of accessible contributions that open the door to this new vision on personal knowledge management.— Prof. Frank van Harmelen——————As the data that individuals need to manage is becoming increasingly complex, there has been a rise in the development of tools and practices to assist in this process. This new generation of tools, although not necessarily based on open and enterprise graph approaches, seem to be converging with them on some level.These tools allow individuals to manage their data as personal knowledge graphs, experienced interactively with edges that can be traversed linking content, in a manner akin to explorations with a “thinking partner”.This timely book thoroughly reviews current research around personal knowledge graphs, with the aim to empower individual users, promoting productivity, data literacy, sovereignty, and interoperability, as well as highlighting future directions.— Prof J. Mark Bishop
- Personal Knowledge Graphs
TODO Foreword - Dr. Ashleigh Faith
It is said that wisdom is built on foundations of knowledge and a road is paved by experience. We have the knowledge part down by now. Knowledge graphs have gone mainstream and are used in everyday applications. An unprecedented amount of data is being created every day but despite this deluge, we lose more data than we gain because of the technological decay of past knowledge vehicles.
지혜는 지식의 토대 위에 세워지고, 길은 경험으로 닦인다고 합니다. 지금쯤이면 지식에 대한 부분은 어느 정도 정리되었습니다. 지식 그래프는 주류가 되었으며 일상적인 애플리케이션에서 사용되고 있습니다. 전례 없는 양의 데이터가 매일 생성되고 있습니다. 하지만 이러한 데이터 홍수에도 불구하고 과거 지식 수단의 기술적 쇠퇴로 인해 얻는 바 보다 많은 데이터를 잃고 있다.
We live alongside digital copies of places, people, and things. Pattern matching and language models are learning billions (and counting) of the quirks of human dialog, the back-and-forth weave of the past with the current, a leap-frog effect of human conversation. Semblances of the human spirit and ingenuity are now composed into prose, art, and digital copies, resurrecting those who have come before and casting thought to what will come. The ethics and boundaries are being drawn, albeit likely much too slow to keep up, and the ideas are being explored, abandoned, and picked back up again.
우리는 장소, 사람, 사물의 디지털 사본과 함께 살아갑니다. 패턴 매칭 및 언어 모델은 수십억 개에 달하는(그리고 계속 늘어나고 있는) 인간 대화의 특이한 점, 과거와 현재의 앞뒤로 얽혀 있는 현재, 즉 인간 대화의 도약 효과를 학습하고 있습니다. 인간의 정신과 독창성을 인간의 정신과 독창성은 이제 산문, 예술, 디지털로 구성됩니다. 사본으로 구성되며, 앞서 온 사람들을 부활시키고 생각하게 합니다. 윤리와 경계가 그려지고 있습니다. 따라잡기에는 너무 느리고, 아이디어는 탐구되고, 버려지고, 그리고 다시 다시 시작하고 있습니다.
But there is a critical component missing if we wish to attain wisdom from all this knowledge. It is experience. The road to wisdom is built from knowledge and paved by experience. Now, supercharged with Personal Knowledge Graphs (PKGs).
하지만 이 모든 지식에서 지혜를 얻으려면 한 가지 중요한 요소가 빠져 있습니다. 바로 경험입니다. 지혜로 가는 길은 지식에서 시작하여 경험으로 포장됩니다. 이제 개인용 지식 그래프(PKG)로 강화 될 것이다.
Personal experiences create a map of each person's accumulated knowledge. Experience is the cycle of experiments, failures, breakthroughs (small and large alike), remixes, revamps and reversals, reconfigurations, and building on what was and what is to come, all to take the next step, and the next. Interpreting and learning from those experiences creates lessons. Lessons shared, passed, and built upon by others create wisdom. And now, personal knowledge can be structured, adding meaning and insights, visible and actionable, owned and operated by you and yours, ready for you to peer into the depths of wisdom that you have, but perhaps were not aware of.
개인적인 경험은 각 개인의 축적된 지식의 지도를 만듭니다. 경험은 다음 단계로 나아가기 위한 실험, 실패, (크고 작은) 돌파구, 재구성, 수정과 반전, 재구성, 과거와 미래의 경험을 바탕으로 다음 단계로 나아가는 순환의 연속입니다. 이러한 경험을 해석하고 배우는 과정에서 교훈이 만들어집니다. 다른 사람들이 공유하고, 전달하고, 쌓아 올린 교훈은 지혜를 만들어냅니다. 이제 개인 지식을 구조화하여 의미와 인사이트를 추가하고, 가시적이고 실행 가능한 것으로 만들고, 여러분과 여러분이 소유하고 운영할 수 있도록 하여, 여러분에게 있지만 미처 알지 못했던 지혜의 깊이를 들여다볼 수 있도록 준비할 수 있습니다.
As Ivo Velitchkov explores in his chapter, breaking down the barriers to innovation and finding new pathways to explore, stones unturned, lessons unmarked are just a few examples where PKGs provide a way to make sense out of the fabric of experiences we all have.
이보 벨리치코프가 이 장에서 혁신의 장벽을 허물고 새로운 길을 찾는 것, 돌이켜보지 않은 돌, 표시되지 않은 교훈은 PKG가 우리 모두가 가진 경험의 구조를 이해할 수 있는 방법을 제공하는 몇 가지 사례에 불과합니다.
There is a tale of Googles-past where there was rumored to be a project to impart, not only knowledge to Google, but also wisdom. The Google Knowledge Graph came to be, but the wisdom part did not, or at least not yet. You see, capturing the web’s data gives you the knowledge, the data points, and how they relate to other things, but not why those matter to individuals or specific situations. That additional interpretation requires the lens of a person’s experiences and circumstances for the knowledge to be passed through, and a way to capture and codify it, which is where tools for PKGs play an important role and which is the focus of George Anadiotis’s chapter.
과거 구글에 지식뿐만 아니라 지혜까지 전수하는 프로젝트가 있다는 소문이 돌았던 구글의 이야기가 있습니다. Google 지식 그래프가 탄생했지만 지혜 부분은 아직 실현되지 않았거나 적어도 아직은 그렇지 않습니다. 웹의 데이터를 캡처하면 지식, 데이터 포인트, 다른 것들과의 관계는 알 수 있지만 그것이 개인이나 특정 상황에 왜 중요한지는 알 수 없습니다. 이러한 추가적인 해석을 위해서는 지식을 전달하기 위한 개인의 경험과 상황의 렌즈가 필요하며, 이를 캡처하고 코드화할 수 있는 방법이 필요한데, 이것이 바로 PKG용 도구가 중요한 역할을 하는 부분이며 조지 아나디오티스가 이 장에서 중점을 두고 있는 부분입니다.
The weave of knowledge and experience depends on interpretation to become wisdom, which is why PKGs, which allow these to become a picture to be assessed and interpreted, supercharge the road to wisdom. Wisdom cannot be contained per se, only insights offered for interpretation, and that by a specific person based on their own experiences. This emphasis on the individual is what makes PKGs different from a traditional knowledge graph. In a PKG, the most important node is the center node representing you as an individual, and the most important edges are the way you interpret the notes to your own personal symphony of life, the weave that makes your world, and how you interpret it.
지식과 경험의 직조는 해석에 따라 지혜가 되므로, 이를 평가하고 해석할 수 있는 그림이 될 수 있는 PKG는 지혜로 가는 길을 더욱 힘차게 만들어 줍니다. 지혜는 그 자체로는 담을 수 없고 해석을 위해 제공되는 통찰력, 그것도 특정 개인이 자신의 경험을 바탕으로 한 통찰력일 뿐입니다. 개인에 대한 이러한 강조는 PKG를 기존의 지식 그래프와 차별화하는 요소입니다. PKG에서 가장 중요한 노드는 개인을 대표하는 중심 노드이며, 가장 중요한 에지는 개인이 자신의 삶의 교향곡에 대한 음표를 해석하는 방식, 즉 자신의 세계를 만드는 직조와 해석 방식입니다.
Each person has lived a life, and interacted with other lives, creating a unique weft of lessons and threads of knowledge that weave into a quilt of wisdom that we can also wrap around the next generation. To comfort. To grow. To guard. To inspire. The most used vehicle for this throughout history has been stories, the narratives to pull together the knowledge and experiences to pass on wisdom to the next. But stories tend to warp, change, take on a life of their own, and unfortunately, they also get lost down the years. Cultural heritage, the stories that make you you, your culture a spice to your everyday life, the way you react and feel, traditions and language, all contained in verbal stories or often outdated formats like tape or paper can now get a second life. These can change by region and family, share themes across cultures, and have versions across the years that change according to the needs of the current generation. Efforts in Canada, Ireland, Kenya, and other cultural heritage and archival organizations have started exploring the use of graphs for saving these narratives.
한 사람 한 사람이 인생을 살아오면서 다른 삶과 교류하고 교훈과 지식의 씨실을 만들어 다음 세대를 감쌀 수 있는 지혜의 이불을 엮어냈습니다. 위로. 성장하기 위해. 지키기 위해. 영감을 주기 위해. 역사적으로 이를 위해 가장 많이 사용된 수단은 지식과 경험을 한데 모아 다음 세대에 지혜를 전수하는 이야기, 즉 스토리였습니다. 하지만 이야기는 왜곡되고, 변화하고, 독자적인 생명을 갖게 되는 경향이 있으며, 안타깝게도 세월이 흐르면서 사라지기도 합니다. 구전으로 전해지거나 테이프나 종이와 같은 오래된 형식에 담긴 문화유산, 나를 만드는 이야기, 일상의 양념이 되는 문화, 반응하고 느끼는 방식, 전통과 언어가 이제 새로운 생명을 얻을 수 있습니다. 이러한 이야기는 지역과 가족에 따라 달라질 수 있고, 문화 전반에 걸쳐 주제를 공유할 수 있으며, 현재 세대의 필요에 따라 수년에 걸쳐 버전이 바뀔 수 있습니다. 캐나다, 아일랜드, 케냐 및 기타 문화 유산 및 기록 보관 기관에서는 이러한 내러티브를 저장하기 위해 그래프를 사용하는 방법을 모색하기 시작했습니다.
As Margret Warren explores in her chapter focused on the role images play in someone’s personal knowledge, PKGs offer a way to contain the interweaving of these stories, the nodes, and the ebbs and flows between different narratives, all forming the backbone of how many of us define ourselves, where we came from, and where we are building to go. The comparison of PKGs to find insights and meaning is not the stuff of scholars alone, but the beauty of the everyday in finding meaning behind the nuances of your life.
마거릿 워렌이 개인 지식에서 이미지가 어떤 역할을 하는지에 초점을 맞춘 장에서 살펴본 것처럼, PKG는 우리 자신을 정의하는 방식, 우리가 어디에서 왔으며 어디로 나아갈 것인지에 대한 근간을 형성하는 여러 이야기와 그 연결고리, 서로 다른 이야기 사이의 썰물과 흐름을 담을 수 있는 방법을 제공합니다. 인사이트와 의미를 찾기 위해 PKG를 비교하는 것은 학자들만의 일이 아니라 일상의 뉘앙스 뒤에서 의미를 찾는 일상의 아름다움입니다.
As Fabrice Gallet and Eduardo Ivanec explore in their respective chapters, the sharing of knowledge and experiences across lives, situations, generations, projects, colleagues, cultures, and disciplines, deriving insights from the interplay of one world view to another, all build to a greater opportunity for wisdom building and sharing, and the creation of these personal connections is often a rich area of study on intentionality and a person’s connection to knowledge and their own personal wisdom they weave, and the good that can be derived from this shared interweaving.
파브리스 갈레와 에두아르도 이바넥이 각 장에서 살펴본 것처럼, 삶, 상황, 세대, 프로젝트, 동료, 문화, 학문에 걸쳐 지식과 경험을 공유하고, 한 세계관과 다른 세계관의 상호작용에서 통찰을 이끌어내는 것은 모두 지혜 구축과 공유의 더 큰 기회로 이어지고, 이러한 개인적인 연결의 형성은 종종 의도성과 지식과 개인이 직조하는 지혜에 대한 연결, 이러한 공유에서 얻을 수 있는 선에 대한 풍부한 연구 영역이 됩니다.
Experiences and their interpretation come with a heavy responsibility as well. Experiences, knowledge, and wisdom all are cultivated by each individual. Sifting through what matters, what can be discarded, or tucked away for another day, building your own mind palace is hard and takes work and time. Fabrice Gallet explores in his chapter that in a way, one might need a set of directions, a guide, or good GPS to find their way through the maze of insights, perceptions, and knowledge to unearth the wisdom beneath the web of connections. A large portion of your personal investment goes into making sense of things and these cannot be so easily auctioned off or mishandled. Wisdom can be misshapen, misinterpreted, used for purposes never intended or well understood.
경험과 그 해석에는 무거운 책임감도 따릅니다. 경험, 지식, 지혜는 모두 각 개인이 쌓아 올린 것입니다. 중요한 것과 버려야 할 것, 또는 다른 날을 위해 접어둘 것을 가려내어 자신만의 마음의 궁전을 짓는 일은 어렵고 많은 노력과 시간이 필요합니다. 파브리스 갈레는 이 장에서 인사이트, 지각, 지식의 미로를 헤쳐 나가 연결망 아래 숨어 있는 지혜를 발견하기 위해서는 어떤 의미에서 방향, 가이드, 또는 좋은 GPS가 필요할 수 있다고 설명합니다. 사물을 이해하는 데는 개인적 투자의 상당 부분이 투입되며, 이는 쉽게 경매에 부치거나 함부로 다룰 수 없습니다. 지혜는 왜곡되거나 잘못 해석될 수 있고, 의도하지 않았거나 잘 이해하지 못한 목적으로 사용될 수 있습니다.
The mantra “my life, my data” has been a flag raised in light of greater access to data, and personal experience is covered under the laws and regulations that govern data gathering, access, and use, such as GDPR. Experiences and wisdom are deeply personal artifacts to life and often are foundations of a healthy and happy life at that. Sharing your experiences and wisdom to help others is a precious gift. Adding the experiences and wisdom of others builds up, grows into something new, and adds odds and ends to help the long and short tails of situations for more specialized coverage of any given topic. But this is why trust and ownership are such critical pillars of PKGs. Writing a blazing message across the sky of your personal struggles, or those of others spells havoc. Throwing a stone into a calm pond just to see the ripples, testing how turbulent you can make the waters, or fooling others into testing those waters, spells distrust. Neither creates a stable foundation to build on, making for a twisted and bumpy road to wisdom and one we may not be able to see the end of if trust and ownership are not maintained and improved. PKGs put ownership on the individuals creating the data. The data is, after all, their own experiences and interpretations, so if there is falsehood, it is at least only a self-serving deception self-contained. These topics have been explored in standards for the semantic web, which is also the theme for Omes Baltes and Maribel Acosta’s chapter.
데이터에 대한 접근성이 높아지면서 ‘내 삶, 내 데이터’라는 모토가 대두되고 있으며, 개인 경험은 GDPR과 같이 데이터 수집, 접근, 사용을 규율하는 법률과 규정의 적용을 받습니다. 경험과 지혜는 지극히 개인적인 삶의 산물이며, 종종 건강하고 행복한 삶의 토대가 되기도 합니다. 다른 사람을 돕기 위해 자신의 경험과 지혜를 공유하는 것은 소중한 선물입니다. 다른 사람의 경험과 지혜가 쌓이고 쌓여 새로운 무언가로 성장하며, 상황의 긴 꼬리와 짧은 꼬리가 더해져 특정 주제를 보다 전문적으로 다룰 수 있습니다. 이것이 바로 신뢰와 주인의식이 PKG의 중요한 기둥인 이유입니다. 자신이나 다른 사람의 개인적인 어려움에 대한 불타는 메시지를 하늘에 띄우는 것은 큰 혼란을 초래할 수 있습니다. 잔잔한 연못에 돌을 던져 파문을 보거나, 물을 얼마나 격렬하게 만들 수 있는지 시험하거나, 다른 사람을 속여 그 물을 시험하는 것은 불신을 불러일으킵니다. 어느 쪽도 안정적인 기반을 만들지 못하므로 신뢰와 주인의식이 유지되고 개선되지 않으면 지혜의 길은 구불구불하고 험난하며 끝을 알 수 없는 길이 될 수 있습니다. PKG는 데이터를 생성하는 개인에게 소유권을 부여합니다. 데이터는 결국 개인이 직접 경험하고 해석한 것이므로 거짓이 있다면 그것은 최소한 스스로를 속이는 것에 불과합니다. 이러한 주제는 오메스 발테스와 마리벨 아코스타의 챕터의 주제이기도 한 시맨틱 웹 표준에서 다뤄진 바 있습니다.
When PKGs are shared or where a PKG is used for critical applications such as health and identity, this is where verification, checks and balances, anonymity, and governance are needed to maintain an equilibrium of safety. What that looks like is still to be determined, but as with any pivotal moment, trust is easily lost and hard to gain. Using the wisdom of past pivots provides us the learnings to test what we share with who and why, and measure it against our trust factors.
PKG를 공유하거나 건강 및 신원 확인과 같은 중요한 애플리케이션에 PKG를 사용하는 경우, 안전의 균형을 유지하기 위해 검증, 견제 및 균형, 익명성, 거버넌스가 필요합니다. 그것이 어떤 모습일지는 아직 결정되지 않았지만, 모든 중요한 순간이 그렇듯 신뢰는 쉽게 잃기도 하고 얻기도 어렵습니다. 과거의 전환점에서 얻은 지혜를 통해 누구와 왜, 무엇을 공유하는지 테스트하고 신뢰 요소와 비교하여 측정할 수 있는 교훈을 얻을 수 있습니다.
Wisdom is not only connected to the interpretations of your past and current situations but also predictions for future decisions. As explored in Martynas Jusevicius chapter, personal knowledge graphs create a window but that is not the main attraction. The appeal is what PKGs help us to do, to know and be known. Knowing without blinders what you have, where it came from, how you obtained or learned something, who has access or the same knowledge or experiences, who can you share that with to gain an accelerated view on a situation, and how these change over time and with different interventions, these all are interpretations to make more informed decisions. Seeing the weave of those experiences can identify holes or focal points, space to grow and explore, where there are errors in your own data or that of others who contribute to or interpret data from your graph. Experiences are often shared with others to gain insights and understanding. Imagine sharing your PKG with your financial advisor so they can guide you straight, seeking a more balanced search experience, or mapping out your cancer survivor journey with treatments, drugs, providers, and support to share with others. Being able to share these things can strengthen your resolve and confidence with others who can guide or support, or help to resolve disputes or incorrect data and interpretations. While these examples are more the promise than reality, the here and now of PKGs, many of which can be explored throughout this book, are setting the groundwork to bring the future innovations and community building to reality.
지혜는 과거와 현재 상황에 대한 해석뿐만 아니라 미래의 의사 결정에 대한 예측과도 관련이 있습니다. 마르티나스 유세비시우스 챕터에서 살펴본 것처럼 개인 지식 그래프는 하나의 창을 만들지만 그것이 주된 매력은 아닙니다. PKG의 매력은 우리가 무엇을 하고, 알고, 알려질 수 있도록 도와준다는 것입니다. 내가 무엇을 가지고 있는지, 어디서 왔는지, 어떻게 얻거나 배웠는지, 누가 같은 지식이나 경험을 가지고 있는지, 누구와 공유하여 상황을 빠르게 파악할 수 있는지, 시간이 지나고 다양한 개입에 따라 어떻게 변화하는지, 이 모든 것이 정보에 입각한 결정을 내리기 위한 해석이라는 것을 눈치 보지 않고 알 수 있습니다. 이러한 경험의 짜임새를 보면 자신의 데이터나 그래프에 데이터를 제공하거나 해석하는 다른 사람의 데이터에 오류가 있는 경우, 구멍이나 초점, 성장하고 탐색할 공간을 파악할 수 있습니다. 인사이트와 이해를 얻기 위해 다른 사람들과 경험을 공유하는 경우가 많습니다. 재정 고문과 PKG를 공유하여 보다 균형 잡힌 검색 환경을 모색하거나 치료, 약물, 제공자 및 지원과 함께 암 생존자의 여정을 매핑하여 다른 사람들과 공유한다고 상상해 보세요. 이러한 정보를 공유할 수 있다면 다른 사람들에게 도움을 줄 수 있고, 분쟁이나 잘못된 데이터 및 해석을 해결하는 데 도움을 줄 수 있는 결심과 자신감을 강화할 수 있습니다. 이러한 사례는 현실이라기보다는 약속에 가깝지만, 이 책을 통해 살펴볼 수 있는 PKG의 현재와 미래는 미래의 혁신과 커뮤니티 구축을 현실로 가져올 수 있는 토대를 마련하고 있습니다.
Sharing and discovering hidden connections is especially interesting to explore for those using PKG data to design and build experiences. There is no such thing as universal wisdom, not really. Each person is unique and therefore while their interpretations may be similar, the interpretations of their specific situations are all unique to them. Those sharing or creating PKGs from personal data can offer experiences that are more granular and intimate than ever before with the help of PKGs, based on similar patterns to bring joy and surprise, delightment and excitement, but as Gregor Rosenauer’s chapter explores, PKGs are not yet mainstream, and therefore their data can be sparse. My grandma or my five-year-old niece do not yet have the know-how to make a PKG. They both fall back on verbal and written sharing, as can be seen in Rosenauer’s depiction of a PKG on a desktop, and not the dynamic representations of PKGs that are so common. So, while PKGs have great promise, there is still opportunity to help everyday folk get into the PKG space and tap the wisdom that might be hidden.
PKG 데이터를 사용하여 경험을 디자인하고 구축하는 사람들은 숨겨진 연결을 공유하고 발견하는 것이 특히 흥미롭습니다. 보편적인 지혜 같은 것은 존재하지 않습니다. 각 사람은 고유하기 때문에 해석은 비슷할 수 있지만, 특정 상황에 대한 해석은 모두 각자에게 고유합니다. 개인 데이터를 통해 PKG를 공유하거나 생성하는 사람들은 비슷한 패턴을 바탕으로 기쁨과 놀라움, 즐거움과 흥분을 선사하는 PKG를 통해 이전보다 더 세분화되고 친밀한 경험을 제공할 수 있지만, 그레고르 로제나우어의 장에서 살펴본 것처럼 PKG는 아직 주류가 아니므로 그 데이터가 희박할 수 있습니다. 제 할머니나 다섯 살짜리 조카는 아직 PKG를 만들 수 있는 노하우가 없습니다. 이 둘은 데스크톱에 있는 Rosenauer의 PKG 그림에서 볼 수 있듯이 구두 및 서면 공유에 의존하고 있으며, 일반적인 PKG의 동적 표현이 아닙니다. 따라서 PKG는 큰 잠재력을 가지고 있지만, 일상적인 사람들이 PKG 공간에 들어가 숨겨진 지혜를 활용할 수 있는 기회는 여전히 남아 있습니다.
New roads traveled are scary and beautiful things, containing possibilities and opportunity, but also uncertainty and the potential for promises unkept. The road to wisdom is no different. There have been many claims to “the next big thing.” And I am not saying personal knowledge graphs are the next big thing either. But they are the foundations for the next big things that are the promises to come. PKGs are not the “thing,” but they are a means to the “thing” that can help pave the road to wisdom, personally and collectively, if we so choose. This book is a compilation of authors who have been journeying in Personal Knowledge for years, all to unravel what is in the box, or at least the possibilities. What will we find? Join us in the next few chapters in exploring how the road to wisdom is being paved today, where it has come from, the open questions and open promises, and where PKGs can serve as a mechanism to build your knowledge blocks and pave your path to wisdom and to hopefully impart and share some along the way.
새로운 길은 무섭기도 하고 아름답기도 하며, 가능성과 기회를 담고 있지만 불확실성과 약속을 지키지 못할 가능성도 있습니다. 지혜로 가는 길도 다르지 않습니다. “다음 큰 것"에 대한 많은 주장이 있었습니다. 저는 개인 지식 그래프가 차세대 대세라고 말하는 것이 아닙니다. 하지만 개인 지식 그래프는 앞으로 다가올 차세대 기술의 기반이 될 것입니다. PKG는 ‘그 자체’는 아니지만, 우리가 선택한다면 개인적으로나 집단적으로 지혜로 가는 길을 열어줄 수 있는 ‘그 자체’로 가는 수단입니다. 이 책은 수년간 개인 지식의 여정을 함께 해온 저자들이 상자 안에 담긴 내용, 또는 적어도 그 가능성을 풀어내기 위해 집필한 책입니다. 무엇을 발견할 수 있을까요? 다음 몇 장에서 지혜로 가는 길이 오늘날 어떻게 포장되고 있는지, 그 길은 어디에서 왔는지, 열린 질문과 열린 약속, 그리고 PKG가 지식 블록을 쌓고 지혜로 가는 길을 닦는 메커니즘으로서 어떤 역할을 할 수 있는지, 그리고 그 과정에서 어떤 것을 전수하고 공유할 수 있는지 함께 살펴보시기 바랍니다.
Fair travels all, and enjoy the ride!
Dr. Ashleigh Faith Researcher in the Knowledge Graph space Founder of Isa DataThing Educational YouTube Channel @AshleighFaith
TODO Introduction : 서론
Edges cross gaps between things not in order to fill them but in order to traverse the space, to reconnoiter the shapes of solid things and draw new lines.
가장자리는 사물의 간격을 메우기 위해서가 아니라 공간을 가로지르며 입체 사물의 형태를 정찰하고 새로운 선을 그리기 위해 사물의 틈을 가로지릅니다.
Curious Minds: The Power of Connection, Zurn and Bassett
What is curiosity? Most likely, you are curious to learn what this book is about, but now you may wonder what curiosity itself has to do with it. A lot, it turns out. Zurn and Bassett (2022) show how curiosity is not about filling knowledge gaps but about making connections. It’s an edgework. It’s graph-shaped. And, as it happens, information, in general, is graph-shaped or has to be when it initially comes in different shapes, and we need a single one to consolidate it.
호기심이란 무엇인가요? 아마도 이 책의 내용이 궁금하시겠지만, 호기심 자체가 이 책과 어떤 관련이 있는지 궁금하실 겁니다. 알고 보니 호기심과 관련이 많았습니다. 주른과 바셋(2022)은 호기심이 지식의 공백을 메우는 것이 아니라 연결고리를 만드는 것임을 보여줍니다. 이 것은 바로 에지워크:이며, 그래프 형태입니다. 그리고 일반적으로 정보는 그래프 모양이거나 처음에 다른 모양으로 제공될 때 그래프 모양이어야 하며, 이를 통합하려면 하나의 그래프가 필요합니다.
호기심 지식의 연결 -> 그래프 형태
Welcome to the first book on Personal Knowledge Graphs! It is still early, and maybe we don’t know enough about PKG yet, but we are certain that this “is a thing” and a valuable one. And we, those that met at this square we agreed to call PKG, came along different streets, searching for solutions to different problems. Some came looking to boost their productivity, some to support their creativity, yet others were on a quest to take back control over their data.
개인 지식 그래프에 관한 첫 번째 책에 오신 것을 환영합니다! 아직은 아직 초기 단계이고, 아직 PKG에 대해 충분히 알지 못할 수도 있지만, 분명한 것은 이것은 “존재"하고 가치 있는 것임을 확신합니다. 그리고 이 광장에서 만난 우리는 PKG라고 부르기로 합의한 이 광장에서 만난 사람들은 서로 다른 길에서 각기 다른 문제에 대한 해결책을 찾으러 왔습니다. 어떤 사람들은 생산성을 높이기 위해, 어떤 이들은 창의성을 지원하기 위해, 또 다른 이들은 데이터에 대한 통제권을 되찾으려는 사람들도 있었습니다.
Knowledge “can be understood as a system of interconnected propositions linked by inferential relations”, wrote Shaun Gallagher (2022) about the ideas of Husserl. “Knowledge graph” then is simply a tautology. Knowledge is a graph. And since persons are those who can know, we had been using personal knowledge graphs way before we coined the term.
지식은 “추론적 관계로 연결된 상호 연결된 명제들의 체계로 이해될 수 있다"고 후설의 사상에 대해 숀 갤러거(2022)는 설명합니다. “그렇다면 ‘지식 그래프’는 단순히 항진식 입니다. 지식은 그래프입니다. 그리고 사람은 앎을 추구하는 존재이기 때문에, 우리는 이 용어를 만들기 훨씬 전부터 개인 지식 그래프를 사용해 왔습니다.
What nowadays is meant by “knowledge graph” is a way of representing knowledge by using only nodes and edges, where nodes represent entities of interest and edges represent relationships between these entities. The benefits of this approach made it spread, first for open and enterprise knowledge graphs, and now for personal knowledge graphs. Some of the benefits are common for all types. Knowledge graphs can absorb complexity. They are flexible and good at handling change. It can be a change of any kind, a change of the environment or an internal change of needs and preferences. This is the special feature of knowledge graphs, technically called “late binding” or, as Dave McComb put it, “schema late”. In other words, you add or extend the schema as you go and when needed, when there is enough knowledge to decide. All kinds of knowledge graphs share this flexibility in space so that they can unify diversity and in time so that they can accommodate change. But personal knowledge graphs have another special feature. They are generators of surprise. They can deliver serendipity on demand.
late binding, schema late
Seneca and Marcus Aurelius kept a certain kind of journal, which may be the earliest examples of “commonplace books” – the first technology for managing personal knowledge. Commonplace books spread and improved and found new ways of being used during the Enlightenment when John Locke wrote an advanced method for organising them. This method spread far and wide. It was used not only during his time but much later by thinkers such as Charles Darwin. But maybe the first technological change towards flexibility was the invention of Thomas Harrison in 1740. His “Ark of Studies” was a wooden cabinet in which file cards were hooked on tin plates. This opening up, together with developing more sophisticated systems of indexing, brought further innovations to the ancient art of excerption and a functional shift, as Alberto Cevolini put it, “from memory aids to secondary memories”. Yet it wasn’t until Niklas Luhmann’s Zettelkasten, when advanced cross-referencing, delayed classification and making unexpected connections reached the level that we now, armed with more advanced technologies, expect from our personal knowledge graphs. For some time, knowledge graphs and personal knowledge management developed in parallel, independently, but recently this has changed. At first, there were only a few graph-based tools for personal knowledge, and then they spread like a virus in the years when a real one showed us that the world is a graph, interlinked more densely than we thought.
And now, in 2023, you have in your hands the first book on personal knowledge graphs. Like any other book, it is a journey. Or rather, many journeys. It’s a personal journey for us, Ivo and George. It didn’t take much courage to start it, we felt we had to, but it took some to keep going and finish it. [ ¹ ] It was a journey for all the other contributors – Eduardo, Fabrice, Gregor, Margaret, Maribel, Martynas and Omes – who joined us. And it will be a journey for you, the reader. We hope it will be a nice walk through new places, maybe a bumpy ride at times, but hopefully, along the way, at some turns, it will reveal alluring landscapes that you would wish to explore further. And depending on who you are and what you are looking for, you may find different things of interest. This is supposed to be a book for any curious mind. Whatever you do, but especially if it involves working with a lot of information, it’s worth knowing how graphs can help. It may be that you’d like to improve your productivity, manage your research better, organise your knowledge so that it stimulates your creativity and regularly brings you surprising connections, or you may simply need a better way to take notes or manage your projects and digital collections. If you are a toolmaker, it might bring new ideas and insights on what you are already working on or inspire you to create something entirely new. And this book is meant to provoke not only by what it brings but by what it doesn’t, the known and unknown gaps to be filled, and the new territories to be explored and connected.
Notes
[1] See https://personalknowledgegraphs.com/#/page/heroes%20journey%3A%20notes%20towards%20a%20pkg%20book Past, present, and future of Personal Knowledge Graphs
Heroes journey: Notes towards a PKG Book
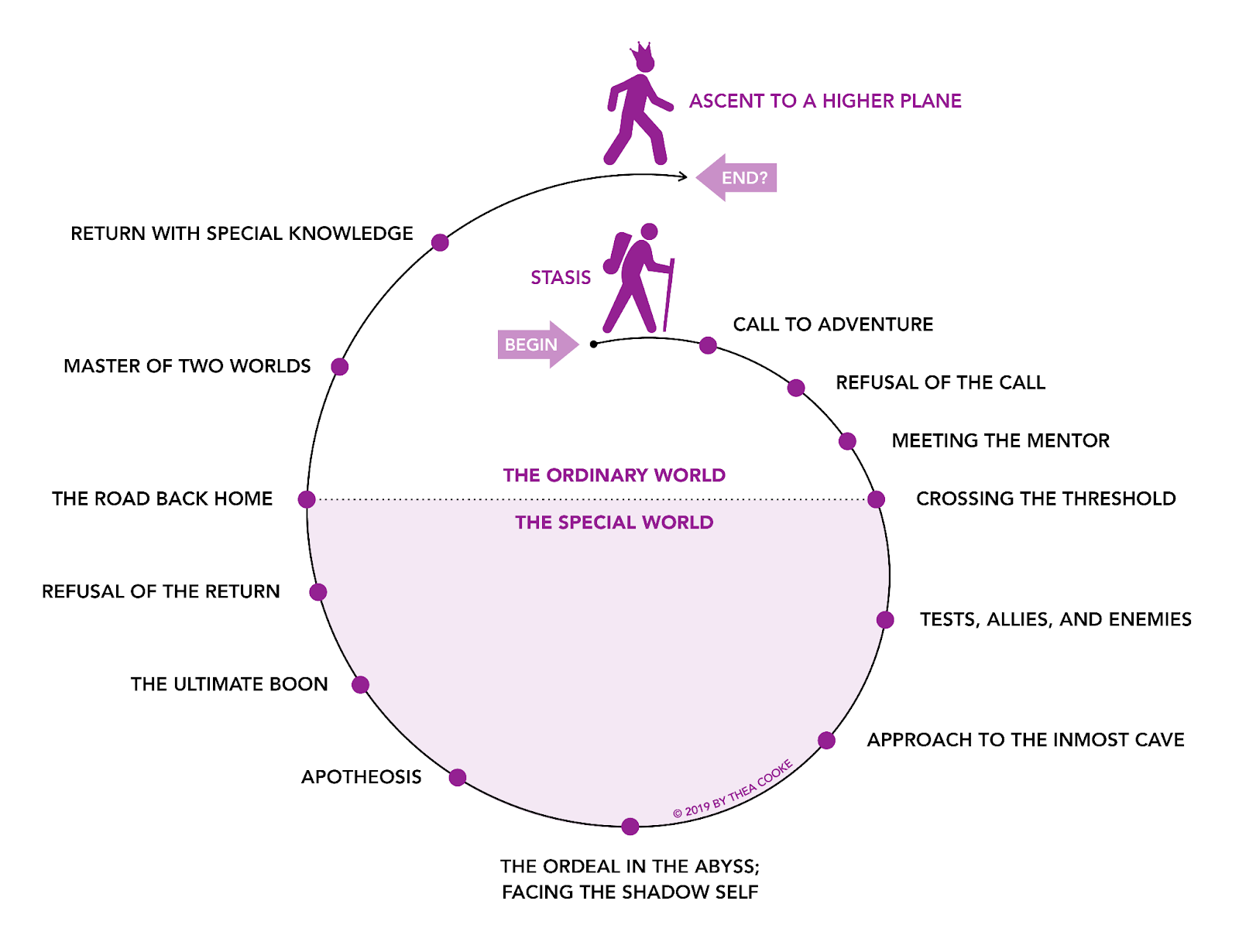
The hero’s journey[1] is a common template of stories that involve a hero who goes on an adventure, is victorious in a decisive crisis, and comes home transformed. Writing a book is like a journey too. The first book on Personal Knowledge Graphs involved more than one hero and a few crises.
Departure
The first part of the hero’s journey is the call to adventure. In this, the hero who lives in the ordinary world receives and initially refuses the call to adventure. Eventually, however, the threshold is crossed and the journey begins.
In our case, the tale began with two heroes. , who came up with the idea for this book, and , who joined Ivo’s calling after initially refusing. Ivo is a consultant, author and speaker who works with knowledge graphs. George is an analyst, consultant, engineer, founder, researcher and writer who also has a background in knowledge graphs.
George organizes Connected Data World, the leading event for those who use the Relationships, Meaning and Context in Data to achieve great things. Knowledge Graphs are a big part of Connected Data World, and Ivo was a member of the event’s program committee in 2021.
In addition, George and Ivo have a keen interest in Personal Knowledge Graphs. George has organized sessions and written on PKGs, and has also explored some PKG tools. Ivo is an avid user and tinkerer of PKG tools and he has also given a few talks on the topic. Both are members of the PKG Working Group, an unofficial group of PKG enthusiasts.
Seeing the growing interest in PKGs as well as the proliferation of tools, Ivo came up with the idea of writing the first book on the topic. He suggested this to George, who initially had to decline. At the time, the preparation for Connected Data World 2021 was in full swing. Organizing an event of this magnitude is more than a full-time job, so the book had to wait.
It wasn’t until the beginning of 2022 that the journey towards the PKG book began. Crossing the first threshold actually encompassed making a number of key decisions and crossing metaphorical thresholds in terms of implementing those decisions.
The first key decision was that the book would be made out of multiple contributions. Even though Ivo and George know PKGs well, they agreed that inviting contributions from different authors would serve two key goals. First, it would add a range of perspectives and use cases, which is very important for an emerging discipline. Second, it would make this a group effort, which is something they both believe in.
The second key decision was about the character and orientation of the book. Neither Ivo nor George wanted to produce an academic textbook. Although they are both familiar with research and have a few publications under their belt, they felt that PKGs have the potential to serve individual users beyond the confines of academia and enterprises. Therefore, they deliberately opened the scope for contributors and readers from all walks of life.
The third key decision was about the book’s medium. That may seem like a paradox – a book is a book is a book after all, is it not? In most cases, it is, but this book about PKGs is different. One of the characteristic features of PKGs and PKG tools is the fact that they enable concepts to be expressed as interconnected nodes and edges that can be browsed on the web. Ivo and George felt that this should be a key feature of their book, which is itself a PKG.
Initiation
The second part of the hero’s journey entails a series of trials and tribulations. In a climactic descent, the hero reaches a low point of (metaphorical or actual) near death, but emerges victorious.
With the first threshold having been crossed, a call for contributions was made public. George and Ivo could feel the excitement as the word traveled far and wide across borders and communities. As the deadline for submissions was closing by, more and more contributions started piling up. On the one hand, that was a first tangible sign of success. On the other hand, it also presented some issues.
First, despite having received a good number of submissions, there were some notable absences too. Most importantly, that of Solid. Solid is a project led by Tim Berners-Lee. It’s a proposed set of conventions and tools for building decentralized social applications based on Linked Data principles, relying on existing W3C standards and protocols. Ivo and George recognize Solid as an important foundation for PKGs and invited contributions from its team, but that did not come to fruition.
Second, evaluating such a wide-ranging set of submissions required careful consideration, deliberation, and hard decisions. Each and every submission was thoroughly vetted in a multi-factorial decision process that involved topic relevance, author qualifications, timeliness, potential impact and more. After a long and laborious process including background research and conversations with authors, an initial list of 15 accepted submissions was compiled.
In parallel, Ivo and George embarked on the task of considering publishers who would be willing and able to take this book under their wing. After a number of discussions, the decision was made to entrust the book to Exapt Press. Exapt Press is a boutique publisher specializing in Systems and Complexity books. Ivo’s previous book, Essential Balances, was published by Exapt Press, too.
This, essentially, meant that from that point on a precious ally was now on George and Ivo’s side. Rob Worth, Exapt Press’s Editor in Chief, became an indispensable member of the team and brought his expertise on board. True to the Hero’s Journey archetype, however, the approach to the inmost cave was only now beginning.
Wanting to be a PKG itself, the PKG book faced another challenge. In order to not enforce the use of any single tool, Markdown was the format of choice. The idea was that collaboration would be structured around a Markdown repository, in this case on Github, that could be used to keep track of contributions and edits. While Markdown and Github work well, collaboration relied on 3rd party tool integrations. That did not work well and had to be sidelined. Cross-PKG tool collaboration does not really work at this point.
In addition, as is to be expected in an effort spanning a few months and involving more than a dozen people, life incidents got in the way in every imaginable and unimaginable way.
George was working on building an eco-friendly house in the Greek countryside and kickstarting an olive oil business in parallel. Ivo had to guide four knowledge graph projects and design and deliver two new training courses. Rob was having disruptive, unexpected and unavoidable repair work being done to his home office for a prolonged period. Contributors also faced a number of issues – from turbulence at work to fighting with personal health disorders.
All in all, that caused the book to fall behind its original schedule and lose some contributions. In Hero’s Journey terms, Ivo, George and Rob went through a period they might as well metaphorically call “the ordeal in the abyss”. Repeatedly reviewing and editing new versions of the contributions, repeatedly interacting with contributors and pushing deadlines, trying to keep sane and productive while juggling tasks.
Reward and Return?
And now? What’s next in this journey? In the Hero’s Journey archetype, if the hero endures through the darkest hour, what comes next is apotheosis and the way home. Are we there yet?
We’re getting there, hopefully. The quality of our contributed chapters has been substantially elevated. Most of our material has gone through the copy editing stage already and is ready for publication.
But, in all honesty, what made us think we’re seeing the light at the end of the tunnel was the most pleasant hard choice we had to make in our journey so far: choosing a cover. The fact that our topic inspired artistic impressions of such high quality meant that we have the privilege of choice.
In addition, sharing those options with the public produced an influx of interest and positive comments. This feedback has strengthened our resolve to push through the finish line. Our reward will materialize by publishing the PKG book. Our return will materialize by advancing our knowledge, and hopefully pushing the domain forward and sharing the knowledge with as many of you as possible.
To be the first to know when the book is out, sign up to be notified here. To learn all our latest news first, as well as be in the know on all things PKG, follow the PKG book on Twitter and LinkedIn.
TODO Chapter 1 Personal Knowledge Graphs – Why, what, and where to?
Ivo Velitchkov
start
The uptake of knowledge graphs is growing rapidly. Three of their qualities make them attractive in various use cases, from open-data publishing through enterprise data integration to machine learning. The first quality is that knowledge graphs can be a universal abstraction layer on top of heterogeneous data structures and so can unify data coming from independent data sources. Second, in semantic knowledge graphs, data, meaning, and rules live together in the graphs making them self-descriptive and potentially independent from applications that use them. And third, knowledge graphs provide flexibility that can help people deal with complex problems while also accommodating change at a very low cost.
Then it is not surprising to see knowledge graphs also enter the field of personal information and knowledge management. And apart from open and enterprise knowledge graphs, there is now a new kid in town: personal knowledge graphs (PKG).
But what are PKGs, and why should we care? Where are we now in adopting PKG, what do we use them for, and how is this likely to evolve?
This chapter tries to answer these and other questions. The first section clarifies what a PKG is, with more attention to the concept of “knowledge.” Then there is a brief overview of the first applications of PKGs. The second section turns to cognitive science to explain why a PKG is not just a tool but also a participant. The third, and largest, section offers a view of the evolution of PKGs, extrapolating to a possible path in the future.
지식 그래프의 사용은 빠르게 증가하고 있습니다. 지식 그래프의 세 가지 세 가지 특성으로 인해 오픈 데이터 퍼블리싱에서 퍼블리싱부터 엔터프라이즈 데이터 통합을 통한 머신 러닝에 이르기까지 다양한 사용 사례에 매력적으로 작용합니다. 첫 번째 특성은 첫 번째 특성은 지식 그래프가 보편적인 추상화가 가능하다는 것입니다. 계층이 될 수 있으므로 독립적인 데이터 소스에서 가져온 데이터를 통합할 수 있다는 것입니다. 통합할 수 있다는 점입니다. 둘째, 시맨틱 지식에서 그래프에서는 데이터, 의미, 규칙이 그래프 안에 함께 존재하기 때문에 자체 설명이 가능하고 이를 사용하는 애플리케이션과 독립적입니다. 셋째, 지식 그래프는 사람들이 복잡한 문제를 처리하는 동시에 사람들이 복잡한 문제를 처리하는 동시에 매우 저렴한 비용으로 매우 낮은 비용으로.
그렇다면 지식 그래프가 개인 정보 및 지식 관리 분야에서도 지식 그래프가 개인 정보 및 지식 관리 분야에도 진출하는 것은 놀라운 일이 아닙니다. 그리고 오픈 기업용 지식 그래프 외에도 이제 새로운 개념의 개인 지식 그래프(PKG)입니다.
그렇다면 PKG란 무엇이며 왜 우리가 관심을 가져야 할까요? 현재 도입 단계 어떤 용도로 사용하며, 앞으로 어떻게 발전할까요?
이 장에서는 이러한 질문과 기타 질문에 대한 답변을 시도합니다. 첫 번째 섹션에서는 PKG가 무엇인지 명확히 설명하며, “지식"의 개념에 더 중점을 두고 “지식” 그런 다음 첫 번째 애플리케이션에 대한 간략한 개요가 있습니다. PKG. 두 번째 섹션에서는 인지 과학을 통해 PKG가 단순한 도구가 아닌 참여자인 이유를 설명합니다. 가 단순한 도구가 아닌 참여자인 이유를 설명합니다. 세 번째이자 가장 큰 섹션에서는 PKG의 진화에 대한 관점을 제시하고, 향후 PKG의 앞으로의 가능한 경로를 추정합니다.
Personal Knowledge Graphs
[T]he basic idea of [associative indexing] which is a provision whereby any item may be caused at will to select immediately and automatically another. This is the essential feature of the memex. The process of tying two items together is the important thing.
Vannevar Bush, As We May Think, 1945
What is a personal knowledge graph?
Answering this question is an act of proposing a definition. Doing it implies that definitions are a good thing. And yet “every definition has a fundamental weakness: It excludes and limits” (Foerster & Poerksen, 2002).
On definitions
Definitions don’t exist out there. They are made by people. So they are personal. Even when an agreement is reached, and a definition is accepted and used by many, it doesn’t stop being personal, it just becomes inter-personal. Definitions are not only made by people but also for people. Much effort is spent carving and agreeing on one definition, while there are different people with different interests and needs and they will be better served by different definitions of the same thing.
Reading a definition lets you know about the thing defined. [ ¹ ] It gives you an idea of what is meant by a word or a phrase, but you don’t automatically get a better understanding. For that, you need to learn about different perspectives, see the term in a range of contexts, and, better still, get firsthand experience. The understanding, then, the living definition, is something that will emerge after some interaction. This interaction can be with PKG tools, books about PKGs, like the one you are reading now, and with other PKG users and researchers.
When using knowledge graphs and ontologies for making glossaries, definitions appear in a fixed state and in a dynamic, networked state. The most popular ontology [2] for creating thesauri is the Simple Knowledge Organization System, SKOS. It has a formal vocabulary, itself a graph, that gives explicit semantics to an instance graph, a thesaurus, or a taxonomy. SKOS has a property [3] skos:definition, [4] that links a node representing a concept to a human-readable definition in a certain language. [5] But more importantly, each concept is linked to other concepts from its own or external vocabularies with semantic relations such as broader, narrower, and related. This way, on top of the interoperability gain, the understanding comes out of knowing not only the definition but also all these relations. Knowledge graphs are open-ended. Adding new edges and nodes is not restricted by a predefined schema. [6] And with the growth of relations, the living definition, the actual understanding, gets richer.
Definitions are like a club with a bouncer at the entrance to check if those who want to enter are qualified. More formally, definitions specify necessary and sufficient conditions. We can define a class of “Personal Knowledge Graph” in an OWL ontology and formally list all the conditions, again as part of a knowledge graph, that need to be satisfied so that a resource having the required characteristics can be classified as a “Personal Knowledge Graph.”
In summary, knowledge graphs themselves have the capabilities to formally [7] define anything, including what is a PKG. Let’s now look for an informal definition of PKG, in natural language.
Since we need to define personal knowledge graph, we need to know first what a knowledge graph is. When the topic of knowledge graphs went viral, just like viruses, the more they spread, the more variants appear, and so there was a great diversity of opinions. In 2019, Michael Bergman found 27 definitions for knowledge graphs (Bergman, 2019) in sources published since 1974. After this divergence, there was an ostensible convergence with the publication of the Knowledge Graphs book (Hogan et al., 2022). There, a “knowledge graph” is defined as:
a graph of data intended to accumulate and convey knowledge of the real world, whose nodes represent entities of interest and whose edges represent relations between these entities.
This definition is clear but relies on a prior common understanding of graph and knowledge.
The concept of graph is easier. It is rigorously defined in mathematics as an ordered triple comprising a set of nodes, a set of edges, and a function mapping each edge to a pair of nodes. It has a well-known history starting with Euler’s negative resolution of the problem of the Seven Bridges of Königsberg in 1736. [8]
But what about knowledge? It seems to be taken for granted. The definition above states “intended to accumulate and convey knowledge.” But can knowledge be stored and transmitted? To answer that, we should get a closer look at knowledge, not with the ambition to define it, but rather to elucidate some aspects and refocus the attention. The term is abused in a way that endows knowledge with characteristics that it cannot possibly have and obscures others that are important both in general and for PKGs in particular.
Knowledge
To define knowledge is beyond the ambition of this section. Instead, I’ll try to bring a few perspectives, one of which unpopular, to shift the understanding from the one that currently dominates in both IT and business circles.
A popular narrative about knowledge, especially in the context of business and information, is that it is something more valuable than information, which itself is more valuable than data. Data, the supporters of that model claim, is meaningless on its own. For example, you see 100, but you don’t know if it is years or dollars or something else. When you learn it’s a measure of degrees, you still don’t know if it’s regarding angle or temperature. And even when you find out it’s a temperature measurement, that cannot bring much information unless you know the units and what is it the temperature of – air, water, or something else. If it is a water measurement in Celsius, then you can connect it with other knowledge and infer that the water is boiling. Sounds convincing and yet it means that information can only be defined in reference to data, and knowledge in reference to information. That is the case at least in the popular data-information-knowledge-wisdom (DIKW) pyramid. What’s worse, it excludes the one and only one who is capable of sense-making, the living individual. I have discussed these issues in more detail elsewhere (Velitchkov, 2017). Now, let’s look at other perspectives, which are not so neat, but are probably less wrong.
According to Husserl, “knowledge […] can be understood as a system of interconnected propositions linked by inferential relations” (Gallagher, 2022). Then knowledge is a graph by definition, so knowledge graph is simply a tautology.
Here is yet another perspective. To inform is to change the state from within. The cause – a temperature change, a poke in the ribs, spoken words, etc. – is coming from irritation received by the nervous system, but the change of state is determined by the structure of the living system and not by the external “message.” Yet, the history of structural coupling [9] produces coordination that an external observer can perceive as a transfer of information from sender to receiver, but nothing like this can possibly happen.
So long as language is considered to be denotative it will be necessary to look at it as a means for the transmission of information, as if something were transmitted from organism to organism, in a manner such that the domain of uncertainties of the “receiver” should be reduced according to the specifications of the “sender”. However, when it is recognized that language is connotative and not denotative, and that its function is to orient the orientee within his cognitive domain without regard for the cognitive domain of the orienter, it becomes apparent that there is no transmission of information through language. It behooves the orientee, as a result of an independent internal operation upon his own state, to choose where to orient his cognitive domain; the choice is caused by the “message”, but the orientation thus produced is independent of what the “message” represents for the oriented.
(Maturana & Varela, 1980)
Beyond biological systems, such understanding of communication has various implications in social systems for explaining, for example, the paradoxical role of decisions in organizations (Luhmann et al., 2018), interactions between organizations (Usher & Whitty, 2017), and the productive misunderstanding [10] between science and industry (Seidl, 2010).
If no transfer of information is going on between biological and social systems, then the same should apply to knowledge. Then the use of “convey” in the cited definition of “knowledge graphs” cannot be understood in the sense of a mechanical transfer. It should be understood in dynamical terms, as something happening when a user interacts with a graph. More on that in the next section.
According again to Maturana and Varela (1992),
the evaluation of whether or not there is knowledge is made always in a relational context. In that context, the structural changes which perturbations trigger in an organism appear to the observer as an effect upon the environment.
This doesn’t define what knowledge is, but when such evaluation is made. It is then relational in a double sense, once to the context of the one appearing to have knowledge and then to the observer making the assessment. Then every behavior can be assessed as a cognitive act and by extension, living, since it is “effective action in existence as a living being” (Maturana & Varela, 1992), is equivalent to knowing.
Knowledge cannot be attributed to nonliving systems or objects. People can know, but graphs cannot. [11] Still, speaking about knowledge graphs is useful. While graphs don’t know, as we’ll see later, there is a good reason to refer to knowledge graphs in that way from the perspective of the extended cognition that they enable. Seen through this lens, even if it is a bit problematic to talk about knowledge graphs in general, it is less so for PKGs. This phenomenon is explained in more detail in the next section. Now, let’s continue our exploration of knowledge graphs by reviewing the main types and some prominent examples.
Types of knowledge graphs
We can distinguish three types of knowledge graphs: open, enterprise, and personal knowledge graphs. Historically first came open knowledge graphs (OKG) such as Freebase, DBpedia, Wikidata, and the Google Knowledge Graph. [12] OKG can be further split into those for general knowledge (or cross-domain) like Freebase, Wikidata, DBpedia, and YAGO and domain-specific ones like Uniprot or EU Publications (CELLAR).
The second type is the enterprise knowledge graphs (EKG). They are implemented as a flexible data-integration solution by unifying data from heterogeneous internal and external data sources or to serve a specific business case for which graph technologies give better results than their alternatives. The two main drivers for enterprise knowledge graphs are the problems coming from the traditional application-centric approach for designing and building software systems and the increased demands of data analytics and machine learning. Many big companies from different industries have implemented enterprise knowledge graphs in recent years. Prominent examples are IKEA, Airbnb, Roche, AstraZenekca, Bayer, UBS, Siemens Energy, Wells Fargo, BMW, Morgan Stanley, and Bosch. An Enterprise Knowledge Graph Foundation (EKGF) has been established recently. EKGF published a set of principles and a maturity model for EKG. [13]
Personal Knowledge Graphs
The third and most recent knowledge graph species are the personal knowledge graphs (PKG). They got some attention from academia and in 2019 Balog and Kenter published a research agenda identifying several areas of future work (Balog & Kenter, 2019). They defined PKG as
a source of structured knowledge about entities and the relation between them, where the entities and the relations between them are of personal, rather than general, importance. The graph has a particular “spiderweb” layout, where every node in the graph is connected to one central node: the user.
(Balog & Kenter, 2019, p. 218)
Since we live in times of mass personalization (Esposito, 2022) it is natural that knowledge graphs are also used for that, so the paper clarified the difference between personalized and personal KG. While the latter inherently enables personalization, it contains a “disjoint set of entities” (Balog & Kenter, 2019) created or curated by the user. These types of graphs have the same acronym and share some capabilities but are of different natures. They get easily confused even in scientific publications. [14]
The paper outlined four research questions. They deal with problems such as knowledge representation, entity linking, graph population, and integration with external sources. Put this way they don’t sound especially new, but they are in the context of the specific challenges when the content is created by and for the needs of one person.
The personal graph population question seems to attract relatively more research efforts with recent contributions for entity and relationship extraction from conversations (Li et al., 2015; Mohanaraj & Laursen, n.d.; Tigunova et al., 2020; Torbati et al., 2021; Yu et al., 2020), from unstructured documents (Vannur et al., 2020) and from heterogeneous data sources (De Mulder et al., 2021; Kalokyri et al., 2018; Montoya et al., 2018). The work of De Mulder et al. has particular significance in two dimensions. Similar to Thymeflow (Montoya et al., 2018), it generates standards-based PKGs with explicit semantics, but in addition, the user has full control over their data, allowing application-data decoupling and fully decentralized architectures. We’ll get back to this point when discussing the evolution of PKGs.
Recommendations seem to be a prominent research topic. The PKG can recommend a diet (Seneviratne et al., 2021), activities (Safavi et al., 2020) or books, recipes, and travel destinations (Safavi et al., 2020).
Overall, it turns out that the current academic interest is in using data that is already created outside the graph, and there is a relatively low intensity of user interaction with the graph, especially in terms of writing and linking. “Personal” in PKG is then used more for knowledge about a person than created by a person. At the same time, in practice, the trends are just the opposite. There is growing adoption of advanced networked note-taking tools, referred to as Tools for Thought (TfT), supporting a wide range of use cases from daily planning and research to project management and design. One way or another many of them treat the user content as a PKG. We’ll review how and to what extent later in the section about the evolution of PKG.
Many new PKG tools are used to support research. That’s not surprising. Luhmann’s Zettelkasten fully qualifies as a PKG (see my other chapter in this volume for details) for research and, as such is the longest used PKG on record – about 45 years. The benefits of its use are evident in the quality and quantity of Luhmann’s work and Luhmann’s own assessment of the contribution of his system and to what extent he relied on it (Luhmann, 1981). [15]
PKGs, or at least those that are used for personal knowledge management, are in a way always already social since the user communicates with her past and futures selves. Once some parts of the personal graphs are shared among different users, we can speak of inter-personal (Lajos, 2019; Ivanec, this volume) knowledge graphs. A closely related category is collaborative knowledge graphs (CKG). Some current PKG tools have collaborative capabilities. However, there can be collaborative platforms based on knowledge graphs that are used only for collaboration, in which case they don’t qualify as PKGs.
After this quick overview of the PKG species and use cases, it’s time to go back and propose a definition. The PKG definition provided earlier from Balog and Kenter is from 2019.
In the meantime, Ruben Verbourgh defined a PKG very inclusively as “All data that you yourself create combined with all data that others create about you.” (Global Data Geeks, 2021).
Recently, Skjæveland and Balog went to the opposite extreme. They revised the definition from 2019, and proposed a stricter one:
A personal knowledge graph (PKG) is a knowledge graph (KG) where a single individual, called the owner of the PKG, has (1) full read and write access to the KG, and (2) the exclusive right to grant others read and write access to any specified part of the KG. The primary purpose of the PKG is to support the delivery of services that are customized particularly to its owner.
(Skjæveland et al., 2023, p. 5)
While this definition is an improvement over the one from 2019 by providing clear criteria and removing the unnecessary condition that facts need to be connected to the user, it skips “knowledge graph”, and focuses entirely on data ownership. Data ownership is indeed an important aspect and central for what I call the “third wave” (see below the section about the evolution of PKG). Yet, “personal” shouldn’t be reduced to only one aspect, that of ownership. I would suggest defining PKGs by a minimal modification of the definition for knowledge graph proposed by Hogan et al. (Hogan et al., 2022), basically removing real [16] and adding personal.
A graph of data intended to accumulate and convey knowledge of the world, whose nodes represent entities of personal interest and whose edges represent relations between these entities.
The graph-extended mind
Natural cognitive systems … participate in the generation of meaning … engaging in transformational and not merely informational interactions: they enact a world.
Francisco Varela, The Embodied Mind, 1991
The simplest information technology is pen and paper. But when we use them what happens is not simple. Once we make a mark [17] on a piece of paper, we start a conversation. It is equivalent to talking in verbal conversation (Glanville, 2007). When we look at the mark to see what it suggests, it is like listening in verbal communication (Glanville, 2007). There is an ongoing interaction, a feedback loop where seeing what we have done is influencing what we think and do next and that in turn modulates the ideas and actions that follow. Importantly, it can help in making completely novel connections that wouldn’t be possible without active involvement with our surroundings.
The engagement between our living bodies and the environment has cognitive significance. Watson and Crick’s work with cardboard models was essential for the discovery of DNA (Watson, 1968). For Luhmann, it was “impossible to think without writing” (Luhmann, 1981, translated by
- Kuehn). The interaction with his Zettelkasten was key for his work.
Then is cognition something that happens within the skull, or is it extended in the environment? Is it a matter of internal processes in the brain, or is it emerging from our interaction with the world?
There is growing evidence that cognition is not a matter of manipulating representations of the external world and is not bounded within the skull but is embodied (Varela et al., 1991), situated (Robbins & Aydede, 2008), extended (Clark, 2003; Clark & Chalmers, 1998), distributed (Hutchins, 1995, 2010), and there is continuity between life, mind (Thompson, 2007), and language (Paolo et al., 2018).
To unpack this a bit, broadly speaking, the main divide in cognitive science is between the computational and the embodied paradigm. The first computational school of thought is Cognitivism, popular in the 1970s and ’80s but still present to this day in different forms. It takes seriously the computer as a metaphor for the mind and sees the brain as something independent from the body and the environment. The brain, cognitivists claim, works by processing internal symbolic representations of a pregiven world. The other computational school, Connectionism, uses the neural network as a metaphor for the brain. It has a more systemic view but still claims that the outside world is only known through internal representations but of a different kind.
In short, according to the computational paradigm, cognition:
Happens in the brain (neurocentrism)
Works with internal representations of the external world (representationalism)
Is disembodied and independent of the environment for its functioning
These three theoretical commitments are in stark contrast with the position of 4E cognition. [18] Some frameworks in this school, such as the extended mind hypothesis (Clark & Chalmers, 1998), distributed cognition (Hutchins, 1995) and enaction (Di Paolo et al., 2017; Stewart et al., 2014; Thompson, 2007; Varela et al., 1991) can help in understanding the interaction of a user with a PKG.
The extended mind hypothesis was proposed by Andry Clark and David Chalmers in 1998 (Clark & Chalmers, 1998). According to that hypothesis, the mind does not reside in the brain and body but extends into the physical world in an action-dependent way. The authors view the engagement of organism and environment as a two-way interaction “creating a coupled system that can be seen as a cognitive system in its own right” (Clark & Chalmers, 1998). [19] Clark and Chalmers give examples with fitting shapes into sockets, writing, playing Scrabble, and ship navigation, referring to the work of Hutchins on distributed cognition (Hutchins, 1995). They also suggest a thought experiment with a hypothetical person Otto, suffering from Alzheimer’s disease, who relies on his notebook the way he would rely on his biological memory if it was well functioning. This example from 1998, involving memory and a notebook, gains additional significance from today’s perspective. In research “the potential for memory support, [is pointed at] as one of the most interesting aspects” (Balog et al., 2022) for application of a PKG. At the same time, in the industry, the advanced applications for networked note-taking are currently the dominant utilization of PKG.
For Hutchins, the question is not where to look for cognition but to avoid setting the unit of analysis in advance (Hutchins, 2010). For some phenomena, the skull can be the right boundary, for others not. His work on distributed cognition shows how many of the cognitive accomplishments attributed to individuals are in fact coming from cognitive systems that transcend individual brains and bodies (Hutchins, 1995) has to be seen as “system of enacted understandings” (Hutchins, 2010). This work is helpful for understanding the interaction between user and PKG and even more for inter-PKG.
Of particular interest here is how the shift to a systemic and socio-technical perspective plays out when what mediates the emergence of mental states is the interaction with complex technological networks like the World Wide Web (Halpin et al., 2013; Smart, 2013; Smart et al., 2010).
Smart et al. (2010) researched the implications of extended and distributed cognition when the extra-neural resources are socio-technical network-enabled environments. The interaction with such environments “does not merely result in the augmentation or enhancement of some well-established ability; it engenders entirely new forms of cognitive processing capability” (Smart et al., 2010). They proposed the thesis of the network-extended mind:
The technological and informational elements of large-scale information and communication networks can, under certain circumstances constitute part of the material supervenience base for (at least some of) an agent’s mental states and processes.
Then the notion of graph-extended mind will not be a matter of implication and extension of this thesis but mere rephrasing. In fact, Smart et al. explicitly point to technologies such as RDF and OWL.
When following this argument, it is possible to interpret it as the mind being extended with objects from the environment, such as pen and paper, a navigation map, or a PKG. To claim that, in a way, these objects themselves constitute the extension. It might even be that some authors are coming close to that understanding. To avoid this risk, it is better to think of that phenomenon as an emergent and enacted mind rather than an extended one.
Another risk is to associate it with the idea of “second brain” that became popular after the courses and the book of Tiago Forte (Forte, 2022). “Building a second brain” is a sticky metaphor and might help in some practices for increasing productivity, but taking it more seriously would require a commitment to two incompatible views, neurocentrism and externalism.
Taking a step further, there is a good reason to believe that such a system, emerging through interaction with certain types of tools, is self-sustained and autonomous, and as such, it’s in the same class of systems as biological and social systems. [20]
What kind of tools can make this happen? Certainly not every tool, and not every software tool. It is a matter of future research to confirm if there are types of capabilities that increase the likelihood of such a cognitive system to emerge. A type of tools that belongs to this class are videogames.
[G]ameplay is argued as being the achievement of dyadic and reciprocal coupling between a player and the game. In this reciprocity, gameplay arises as autonomous organization that is both self-sustaining and precarious. (Vahlo, 2017)
And most likely another type of such tools are PKGs. Already Luhmann admitted, that after some extensive work with his Zettelkasten, what arises “gets its own life, independent of its author” (Luhmann, 1981) [21]. The more advanced technologies available now can accelerate that process.
The viability of such emergent systems can be analyzed through the lens of a small set of structural balances, like the one between autonomy and cohesion (Velitchkov, 2020). These systems don’t exist in a vacuum but are dependent on the viability of the socio-technical system they are embedded in. But since these socio-technical systems are of the same class, their viability can be analyzed by the same set of balances.
Evolution of PKGs
What is the current adoption of PKGs? How will it change, and how will the PKG evolve?
A question such as “Who is currently using a PKG?”, depending on the interpretation, can have two extreme answers:
Nobody is using a PKG yet.
Everybody is already using a PKG.
If we apply certain qualification criteria for the current state of play will give an answer close to the first extreme. If we use the same criteria, we can expect, slowly or quickly, to move to the second extreme in the future. But depending on how a PKG is interpreted, both answers can provide a fair description of the current state. More importantly, this interpretation shows an interesting trajectory of how the ecosystem is evolving.
The First Wave
Mere compression, of course, is not enough; one needs not only to make and store a record but also be able to consult it.
Vannevar Bush, As We May Think, 1945
Since the dawn of personal computing, certain clusters of needs, real or first only imagined, crystalized into application classes such as text processing, spreadsheets, and presentation programs. Being conveniently packaged solutions made them popular and in demand, which in turn further improved and solidified them. Our life got better, but its digital extension grew highly fragmented.
If we look at what types of data we work with, we see that each one is only managed by its dedicated application. We keep our bookmarks in a browser or in some web application for annotations. We create documents with our text processing application, but from there, while we write, we cannot search for our bookmarks. When we share a document with another person, they have difficulties with it unless they happen to use the same text editor. [22] Our task lists are separated from bookmarks and documents. Some applications offer a combination of mail, task, and calendar management, but if we have a more sophisticated way of planning our days, we opt for dedicated a task management application. Similar integration is often featured in traditional note-taking applications. They may offer a web-clipping capability bringing integration of notes, bookmarks, and annotations. Yet we have a separate application for managing projects, yet another for collecting research papers, one for spreadsheets and viewing photos, and one for databases. Our personal data is disconnected. Each application and each document is a silo. But apart from fragmentation, personal information management tends to suffer from digital hoarding and re-finding problems.
The easier it was to create and store photos, bookmarks, and notes, the more we collected. Gradually we turned into information hoarders. It is often that we find something valuable to keep at the moment, and we never use it later either because we don’t need it, we forget about it or we simply cannot find it. Thus, we can broadly distinguish four cases:
Something we believe is valuable at the moment we collect it, but it never actually is;
Something that’s actually valuable, but we have trouble finding it when we need it;
Something that’s valuable, but we forget we have it;
Something that might be valuable in a certain context, especially if it appears there (semi)unexpectedly.
Some of these cases are easy to see in web foraging. We want to go back to something we have assessed as valuable. And one of the strategies to do so is bookmarking. It is the fastest method to refind websites (O. Bergman & Whittaker, 2016), and yet a recent study shows that it’s practiced only by 16% of the study sample, with the rest preferring alternative strategies (O. Bergman et al., 2021) like orienteering (Teevan et al., 2004) [23] or reenacting their first experience with the information they are trying to refind (Jones et al., 2014).
Different strategies for refinding [24] are applied depending on what is searched, [25] but each type of digital object is accessed via a separate tool or a separate way within a tool. We look for files using the operating system’s file manager. We look for web documents using the default search engine in our browser [26] but then use a different search method to find bookmarks. [27] When we are in the same document, for example, Microsoft Word, we are not able to look for information from other types of documents like presentations and spreadsheets.
The following situation is common. We get a question from a friend about a specific topic, and we happen to know of a good book that addresses that topic, but we can’t remember the name of the book. Since we don’t have the book name, searching for the topic and something associated with it doesn’t help. Then we remember it was recommended by another friend, but we don’t remember on which channel, so we go and check the messages with that other friend on email, Messenger, Signal, and Telegram. We don’t find it. What we find instead, luckily, is another message that prompts us to check the text messages on the phone. And finally, there it is. A similar common case is when a file was shared, and we can’t find the link, but we remember the context – it was at a meeting – and so we search in our calendar in hope to find it in that meeting invitation.
All these different types of items, email, contact, calendar event, photo, web address, highlight, paragraph, slide, to-do item, and project issue, are valuable in the network of relations. And there are different types of relations. Some relations are between items, like a paragraph in an email is related to a bullet in a slide deck. Other relations are between the items and the context they were created in or otherwise first experienced in. Probably most important are their relations to new contexts, where they can bring value if only we can easily get to them.
And we do, to the extent to which we can keep these links in our heads.
That’s why we can say that everybody uses a PKG already.
But that kind of PKG is a precarious structure. The nodes live trapped in application silos and files. We keep the edges in our heads or construct them on the fly by reenacting the previous encounter or by taking small steps to narrow down the possibilities.
When seen through the lens of the autonomy–cohesion balance, our personal information system has a lot of cohesion within applications. We are highly dependent (reduced autonomy) on those we use because we like them or because the migration is costly. At the same time, our digital environment doesn’t provide cohesion for our data. Instead, we provide some cohesion ourselves but only to the extent allowed by our memory and by our tolerance for manual actions.
I’d suggest calling this first wave of PKG, quazi-PKG. The nodes are stored, but they don’t have global identifiers and can only be used from a dedicated application. Another application is not able to provide the same functionality even to a resource of the same type. The edges are not stored electronically. We keep them or construct them following some chain of associations with or without the help of various cues from our physical or digital environment.
The wave of the quazi-PKG shows a deficiency in personal computing. It is not surprising that some of the efforts of the following waves apply PKG to address exactly this deficiency (Montoya et al., 2018; Obenauer, 2021; Safavi et al., 2020; Rosenauer, this volume).
The Second Wave
Every note receives its quality only from the network of links and back-links within the system.
Niklas Luhmann, Communicating with Slip Boxes, 1981
The world gets more complex and unpredictable. There are more stimuli, both in number and variety, and they need to be balanced with matching responses (Velitchkov, 2020). Dealing successfully with the world depends on having requisite variety (Ashby, 1958/1991, 1956/2015). That can be one plausible explanation for why in the period 2020–21 there was an eruption [28] of tools for networked note-taking, often referred to as “Tools for Thought.” Just like the new virus variants kept emerging and spreading, new variants of TfT popped up almost every month, some attracting lots of users and creating whole ecosystems in no time. Besides users with no additional involvement, the ecosystems include bloggers, newsletter writers, people making videos with tips and tricks, trainers, contributors, and developers of extensions and themes. Many of these TfTs treat the data, in one way or another, as a knowledge graph.
The birth of this new market went in parallel with increased interest in PKGs by academia, with little if any influence between the two.
This is the second wave, which I suggest calling proto-PKG. [29]
Do these tools qualify as PKGs? We can’t give a simple yes/no answer. Instead of Boolean, we should apply fuzzy logic here, where some tools are more members of the set than others.
Tools such as LinkedDataHub (Jusevičius, this volume), ImageSnippets (Warren, this volume) are in the center, along with Thymeflow (Montoya et al., 2018) and the other research prototypes mentioned earlier in this chapter. The place of Codex, “a text-as-graph solution that integrates a text-as-graph meta model via standoff property annotations” (Palladino et al., 2020) using Neo4j, and ixnote using a proprietary graph DB written in Swift, is in the center too. Very close to the center is Kanopi. It doesn’t use RDF or LPG technology, but conceptually implements the RDF model on top of PostgreSQL. It can pull RDF from websites, and then translate it into the internal data model. The database design does not create schema dependency by using a table for each entity type. Instead, there are only [30] two tables, one for nodes and another for edges.

Figure 1.1 Data model of Kanopi (simplified)
A bit further from the center is a family of TfTs, sharing a similar approach, architecture, technologies and capabilities but having different backend and business model. The family includes Roam Research, Logseq, Athens Research, [31] and Hulunote. They are all written in Clojure, use Datalog for querying and Datascript as a client-side database.
Since all in that family followed the pioneering work of Roam Research, having a quick look at its data model will be sufficient to show why all of them qualify as PKG tools.
There are two main types of nodes in the graph that can be related with :block/children relationship. [32] One is called “page” and in the UI serves as a container of “blocks,” which is the second type of node. Block nodes can have the same type of relationship with other nodes, appearing in the UI as a hierarchy of nested blocks. The actual content of a block is a string, and can be seen as another node type, along the relationship :block/string. This string, the content of a block, can contain references to both pages and nodes.

Figure 1.2 Data model of Roam Research (simplified)
Another reason to consider this set of tools close to the center of the PKG set is that they use Datalog. Datalog is a declarative programing language and its graph querying capabilities are similar to SPARQL. The basic query patterns are even syntactically similar: [?s :block/children ?o] (Datalog) and {?s :hasChild ?o}(SPARQL).
The data structure of tools like Roam Research and Logseq can be generalized as a hyper-graph, from the perspective of “pages”, where blocks play the role of hyper-edges (see figure 1.3).

Figure 1.3 Block-centric TfTs like Roam Research and Logseq, can be generalized as hyper-graphs.
The tools in the Roam family are clearly PKGs, yet a little away from the center of the set. While users have a lot of flexibility when working with nonstructured and semistructured data, they are not able, for example, to specify types of nodes and edges and overall don’t have a way to provide more explicit machine-processable semantics. It can be only partially achieved through combinations of conventions and rules [33] or through extensions. [34] The PKG tools in the center of the set can provide explicit semantics via RDFS/OWL ontologies (De Mulder et al., 2021; Seneviratne et al., 2021), “aspect-oriented” ontology (Palladino et al., 2020), or in other ways. The PKG tools from the second group (and from the third) don’t allow the use of external ontologies at the moment and don’t provide ways for the user to create and use their own. It is often deemed unnecessary and as an additional overload. Yet tools like Tana and Capacities proved otherwise.
Moving to collaborative and interpersonal knowledge graphs – and some tools in this group provide such capabilities already – the lack of ontologies or other means for providing shared meaning limits the potential of coordination and querying. In addition, it limits the effective reuse of content created outside the collaborative space, either in PKGs of the users or from OKGs.
Further from the center of the PKG class are Markdown-based tools like Obsidian, Foam, Dendron, Zettlr, nb, Emanote, Cosma, Bangle.io and Nota. What they have in common is their use of local Markdown files. [35] These tools can be seen as having a conceptual graph model where each Markdown file is a node connected to other files. The connections are created by the user in the text of the file using the common syntax of wikilinks: double square brackets. Each tool provides a way to keep node metadata, often encoded as front-matter YAML, an approach pioneered by Jekyll. Some of these tools, such as Obsidian, allow the user to identify and refer to blocks within the text in a similar way as the second group. Yet, all these applications are further away from the center of the PKG set for two main reasons. They don’t have as much “structured knowledge” (see the definition of Balog and Kenter, quoted in the second section) as the previous groups and graph granularity (the graph is at the level of the pages, not paragraphs), and they don’t treat relationships as a first-class citizen.
Apart from these three groups, there are other tools that can be regarded as PKGs, most of them distant from the center of the set. They include old ones such as Cmap [36] and The Brain and newcomers like Heptabase and Scrintal, where the visual graph is not a matter of a view over the textual input but is how the user creates the content.
What are the main achievements and potential benefits of the second wave?
Unlike the first wave, many of the relationships between ideas and other personal data items are explicit. The cohesion is increased but not at the price of too many restrictions: there are affordances providing flexibility so that the user can both exploit and explore [37] their graphs in different ways, adapted to their work styles. These PKG tools support use cases such as learning, journaling, research, nonlinear writing, project management, personal relationship management, time planning and logging, ideation, collaboration, recommendations, meeting management, and many more. The ability to reuse the same node in a different context [38] and to resurface it at a different time provides additional benefits from the interaction between the user and their graph.
This generation of PKGs has brought many unique benefits already and has the potential to bring more. Working with PKGs cultivates new working and thinking habits. Now we even talk about “graph-thinking” (Gosnell & Broecheler, 2020). Thinking in graphs may help in dealing with complex problems (Nathan, 2021), it can contribute to the adoption of EKG and Linked Data (Velitchkov, 2021a) and it leads to exapting [39] of features of some non-graph tools so that they are used as if they are graph tools [40] and creating extensions providing graph-like experience. [41] A proliferation of such practices may lead to the evolution of these tools towards knowledge graphs.
What are the limitations of the PKGs in the second wave?
These PKG tools are more inviting to create interconnected personal data and to integrate more of the ones created outside the tool. Yet, even with them, most of our personal data is still outside the PKG, residing in emails, spreadsheets, and slide decks or in social media platforms, lifelogging and healthcare data stores. For example, if I want to search for “PKG” in my personal data, I would like to get relevant results from my text documents, PDFs, email, eBooks library, conversations in LinkedIn, Twitter, Telegram, Signal, Discord, and so on. With the current tools, where the PKG is coupled with the application, and the external ones are using their own storage, I can’t.
In that sense, “Nobody is using PKG yet.”
The origin of all these limitations of the second wave is that the current PKG tools are predominantly application centric. They neither replace the functionality of other applications for personal data or the services of the collaborative platforms, nor those applications and platforms that use storage controlled by the users. What is needed is more flexible, person-centric ways of achieving interoperability (cohesion), while allowing freedom (autonomy) for choosing and combining applications and services managing personal data.
The Third Wave
Data is self-describing and does not rely on an application for interpretation and meaning.
Data-centric Manifesto
A good name for the third would simply be “PKG” but if we have to pick a prefix, like it was for the other two, it will be “d-PKG.” That letter “d” can suggest any of decentralized, de-coupled, and data-centric, or all of them.
For the third wave, there are signs and factors coming not just from the evolution of PKGs, but also from the historical trend for increased decoupling in personal knowledge management (see the section “Decoupling” in the second chapter), and more generally from the growing discontent with the current state of the web and enterprise IT. If the third wave is going to happen the way it is envisaged here, it will be due to the interplay of all these factors, so it is worth sketching the larger context.
The web was designed to be a decentralized system where the agreement on a few standards, basically HTTP and HTML, enabled free choice on just about anything else. People were finally free to express themselves and to choose from where and how to get information. They were free to innovate on building new browsers, websites, and whatever web applications and services they could think of. A system like this, with a self-maintained organization, can work well and has a natural tendency for virtuous cycles. In other words, it can amplify goodness and develop its own immune system for whatever threatens its viability. All it needs is to have the right kind of enabling constraints, for example, the standards mentioned above, and to allow autonomy of all subsystems. This is again the balance between autonomy and cohesion (Velitchkov, 2020). It works for animals, people, tribes, organizations, society, and for socio-technical systems like the web.
And the web flourished as a decentralized system where people were free to choose and create more choices. And then, one day, the platforms appeared. They offered good services for free. Or at least they looked good and free at first. In reality, they were (and are) neither good nor free. The platforms are not nearly as good information providers as the decentralized web before them. What we see is not what we are looking for but what their algorithms decide [42] to show us. And the services of these platforms are not free. Quite the contrary. In fact, we pay twice. Once by being their content providers and a second time by giving them our personal data. Importantly, we don’t give them only our current personal data but also future data by allowing them to track our online behavior. In this way, the web, a decentralized system, shaped by the users, turned into a hyper-centralized [43] system, shaped by a few powerful corporations. It also formed users’ expectations. In 2019 Facebook and Google announced that it was now possible to copy images from Facebook to Google Photos. That’s the new norm for innovation. As Ruben Verborgh pointed out, 50 years after being able to send video signals over a distance of 380,000km, we celebrate that we can finally move a photo by 11km [44] (Verborgh, 2020).
Centralization may be beneficial for the internet in a few cases, [45] but when it comes to the layer of content and services, it creates global damage ranging from limiting pluralism and competition to suffocating innovation (Thierer, 2016; Verborgh, 2020), spreading fake news, and manipulation of society (Cadwalladr & Graham-Harrison, 2018; Christl, 2017; Zuboff, 2019; McNamee, 2019).
In enterprises, for decades applications were built in a way where the data model is separate for each application, trapped inside it, and the interpretation of the data is in the application code. This led to a high cost of change and a high cost of data integration (McComb, 2018). Such a traditional, application-centric way of building applications is dominant to this day. Most big enterprises have thousands of application silos. They try to integrate the data through data warehouses, data lakes, point-to-point interfaces, and APIs. All these methods provide partial and temporary solutions and add to the technical debt.
What is common in these problems, on the web and in enterprises, is that data is tightly coupled with applications and platforms. Hence the movements toward decoupling, best captured by the data-centric principles (Data-Centric Manifesto, n.d.):
Data is a key asset of any person, organization, and society.
Data is self-describing and does not rely on an application for interpretation and meaning.
Data is expressed in open, non-proprietary formats.
Access to and security of the data is a responsibility of the enterprise data layer or the personal data vault, and not managed by applications.
Applications are allowed to visit the data, perform their magic and express the results of their process back into the data layer.
The movements for web decentralization bring their own set of principles, to “urge coexistence and interoperability, and discourage walled gardens”. [46] Striving for interoperability is in line with the third principle of the Data-Centric Manifesto and with the FAIR principles. [47]
It seems the same pattern of tight coupling between data and application, platform or host, is manifested in all levels. The “walled gardens” (Berners-Lee, 2009) in the web are like the application silos in enterprises and like the personal applications and files from the first wave, as well as most of the PKG tools from the second wave.

Figure 1.4 From silo apps and files, through powerful PKM systems, to decoupled PKG, fully controlled by their owners
A somewhat uniting theme for the third wave then is decoupling. [48] The focus is decoupling application and data, but also decoupling between host and content (Jacobson et al., 2012) and decoupling of functionalities:
Instead of needing to pick a single email client, can I compose my favorite email client out of an inbox, a compose window, and a spam filter?
(Litt, 2021)
What will PKG look like in the third wave? Here we can finally talk about PKGs by themselves and not about tools for personal knowledge management that use knowledge graphs in some way (the second wave). It might not be a single but distributed storage, yet with a coherence bringing the same experience as if it’s in a single store. In any case, the data is fully owned and controlled by the user, allowing application components to use and manipulate it in a way controlled by the user. This will also enable platform-, application-, and possibly also host-independent inter-PKGs.
What are the current signs the third wave is starting?
One sign is the Solid project, which is a prime example of PKGs in the third wave. In a Solid architecture, the user PKG resides in store providers called “pods”. Users freely choose the pod providers and can grant access to different applications. There are still many challenges, but it seems that the project is going beyond the research and prototyping phase and there are some reports of productive use (Social TV and the Future of Data, 2022). The recent decision of the Flemish government to provide Solid pods to citizens (The Flanders Government and Solid, 2020), if successfully implemented, might further accelerate the development of the needed technologies and wider adoption.
A second sign is coming from the current trend for local-first PKM tools. The majority of those are Markdown-based tools for networked note-taking. Although it can be argued that Markdown is not a real standard, exists in many flavors and has multiple issues (Melvær, 2022), it has wide adoption that goes beyond the developer community. All tools of the third group in the second wave use Markdown, following the local-first principle. This way, the content of users, save for some syntactic differences such as block IDs and document metadata, is independent of the application. It can be viewed and manipulated from one application, say Obsidian, and then the user can continue editing with Logseq or Foam to use a specific feature. This is already a case of data-application decoupling and users having full control over their data. Logseq went a step further, providing a way to query the data independent of the Logseq application, and to convert the graph to RDF. [49]
And the third sign is the shift from functionalities provided by the core application toward extensions and plug-ins. As is often the case, certain technology trends can be seen from what first appears to be useful to the technology professionals. It can be speculated that the way such an ecosystem evolves can signal an overall trend. If we take, for example, VSCode, [50] the data there is decoupled from the application, can be used with another editor, and, more importantly, developers can collaborate using their tool of choice – a great autonomy that is balanced with the cohesion provided by Git. And then many functionalities – for some people, the crucial functionalities for their work – are provided by extensions. More importantly, many extensions seamlessly use the capabilities of others. A similar situation is now observed with the growth in number, sophistication, and adoption of extensions for tools such as Obsidian, Logseq, and Roam.
A fourth sign is the development of protocols such as the Block Protocol [51] and Noosphere, [52] and the appearance of services such as Agora (Ivanec, this volume) and Samepage, [53] which provides seamless integration of content created using independent TfTs.
A fifth sign, or rather enabler, is the progress with various decentralized technologies such as content-addressing (Benet, 2014), distributed ledgers (Zichichi et al., 2020), decentralized identifiers (Decentralized Identifiers (DIDs) v1.0, 2022), and more concretely the first attempt of using Solid over IPFS (Parrillo & Tschudin, 2021), and recently Noosphere, described as a “protocol for thought,” and “worldwide knowledge graph on top of IPFS” (Brander, 2022).
Conclusion
Knowledge Graphs are coming full circle. Conceptually they appeared to solve the problem of organizing information at a personal level (memex, Zettelkasten), then in practice at the organizational level (the first Hypertext systems), after that at global (WWW, and then LinkedData and Google Knowledge Graph), and then back to organizational (Enterprise Knowledge Graphs) and now back to personal (PKG).
Today OKG, EKG, and PKG coexist, and the potential synergy is enormous. OKGs and PKGs can enrich each other. The success of the first EKGs can boost the use of PKGs, which in turn can help people start thinking in graphs and turn to knowledge graphs more often to tackle data-related challenges inside and outside organizations.
In a way, everybody is using a PKG already, but while most of the nodes are digital, most of the edges are mental. This is how we used to provide cohesion to our digital world, but recently we have started outsourcing that cohesion to PKG applications. Next, cohesion has to be provided by technologies and protocols, allowing control over our data and, at the same time, freedom to choose and combine applications, capabilities, and infrastructure. This will allow more innovation that will increase the power of our graph-extended minds to solve complex problems.
Notes
[1] Sometimes it lets you know more “about the person supplying the definition” (Foerster & Poerksen, 2002).
[2] In computer science and information science, ontology is defined as “an explicit specification of a conceptualization” (Gruber, 1993). It usually encompasses the representation, formal naming and definition of entity types (classes), relationship types (properties) and logical axioms or rules that define the consequences of the intended meaning of the ontology terms within one or more domains of discourse. Unlike data models, which are often specific for a certain context, application or organization, ontologies can be used by many applications and organizations, and can be created in one context and used in another.
[3] In RDF, the term “property” is used both for relation between two things, in other words for the meaning of an edge between two nodes, and to link a thing to a simple literal value, such as number, date or a string.
[4] But interestingly skos:definition is a subproperty of skos:note. I choose to interpret this as rightful demoting of the status of definition. The functional benefit is that when one queries for all values of skos:node, in other words, all nodes that the directed edge identified with skos:node points to, this will potentially provide, besides definition, also examples, scope and history notes. They can be retrieved either by a SPARQL engine or by an inference engine that will apply the sub-property axiom and will bring the values of the subproperties of skos:note as inferred values of skos:note.
[5] The use of SKOS and any other RDFS or OWL ontology allows decoupling between the identity of the resource, represented through an URI, and its human-readable label. There can be many labels, one per language, linked to the same URI thought the same property, which is just another URI. When there is more than one label per language, it is better to use a different properties for the different types of labels like it is the case with skos:altLabel and skos:hiddenLabel.
[6] Within relational and object-oriented paradigms, the schema needs to be defined in advance. To store something in an SQL database, a table needs to be created for that type of thing. To create a new object, there needs to be a new class. In knowledge graphs the type of a thing is not linked to the storing mechanism. It can be added later and can be more than one. In the case of RDF graphs, the type is just another node in the graph. The same possibility to postpone definitions applies for defining attributes and relations. This distinguishes semantic knowledge graphs from systems requiring schema-on-write or schema-on-read.
[7] Here “formally” refers to definitions that are unambiguous for both humans and machines.
[8] The story goes that it was a popular weekend activity to try to walk over every one of Königsberg’s seven bridges, which linked islands in the river, crossing each bridge only once. Euler showed mathematically that this task was not possible, thus laying the foundation for graph theory, anticipating topology and spoiling Sunday walks for the good people of Königsberg.
[9] Structural coupling is the “a history of recurrent interactions leading to the structural congruence between two (or more) systems” (Maturana & Varela, 1992).
[10] According to Luhmann, social systems reproduce on the basis of their closed network of internal communications. Science is one such social system which works by its internal true/false code. The economy as a whole is a different societal subsystem, and so is every organization. Applying science in industry is a matter of communicating across a system boundary. Such communications are only a perturbation that can trigger internal communication in systems outside science according to their own code and references. As such, it is inevitably misunderstood anywhere outside science. Yet, scientific communication can have relevance in the industry. In those cases, the misunderstanding is productive.
[11] If we ask (send a query to) a graph and we get a good answer, we can say that graph knows the answer but that will be an anthropomorphic metaphor. Such an anthropomorphic pattern is common not only for knowledge graphs. It appears when people talk about large language models that react on prompts in natural language. But there the answer – as impressive as it may be – is not even a matter of information retrieval. It is just a generated predication of an answer, “bypassing” semantics, understanding and common sense.
[12] The Google Knowledge Graph is a publicly accessible knowledge graph but it is not as transparent as DBpedia and Wikidata. Its new API is on https://cloud.google.com/enterprise-knowledge-graph/ which is yet another reason to treat is somehow between OKG and EKG.
[13] See https://www.ekgf.org
[14] For example, Seneviratne et al. refer to the Personalized Heath Knowledge Graph case of Gyrard (Gyrard et al., 2018) as “Personal Knowledge Graph” (Seneviratne et al., 2021).
[15] I used the translation of Manfred Kuehn (Kuehn, n.d.).
[16] Here, probably only “real” creates some unease. After all, a graph describing Disney World should be a proper knowledge graph and still not being about “the real world”. To which, Juan Sequeda, one of the authors, made the following clarification on Twitter
Real world refers to the domain of discourse of a user. If the “intangible thing” is what you care about, then it’s part of your real world.
Yet, I’m not convinced of the value that adjective adds in the definition and I suggest dropping it, at least when it is reused to define a PKG.
[17] Making a mark is an indication of distinction which by itself is the atomic cognitive operation. This realization by George Spencer-Brown and the calculus of indications developed by him (Spencer-Brown, 1979) across disciples including biology (F. Varela), mathematics (L. Kauffman), social sciences (N. Luhmann) and computation (W. Bricken).
[18] 4E stands for the overarching claim that the cognition is not only in the head but is also embodied, embedded, enacted, and extended. The 4E cognition can be seen as the third school of cognitive science but is in fact a broader movement where different schools such as extended cognition and enactivism, although having in common their opposition of the theoretical commitments of computational paradigm, have significant differences.
[19] The embodied cognition in general is heavily influenced by the theory of autopoiesis by Maturana and Varela, and they defined structural coupling as “history of recurrent interactions leading to the structural congruence between two (or more) systems” (Maturana & Varela, 1992).
[20] Apart from biological and social systems (De Jaegher & Di Paolo, 2007; Paolo et al., 2018), this seem to be the case also for emotions (Colombetti, 2014) and habits (Egbert & Cañamero, 2014).
[21] This phenomenon is explained in the chapter “The Personal Knowledge Graph of Niklas Luhmann” in this volume.
[22] The only mass application that achieved some level of interoperability was email. We can use one application to write an email and the addressee can open, read and reply to it with a mail client of their choice. An even better level of interoperability was achieved by editors for code developers, the so-called IDEs like VSCode but that is a specific and not a mass market case.
[23] Orienteering is defined as “series of small steps were used to narrow in on the target” (Teevan et al., 2004).
[24] Long before digital technologies appeared, Vannevar Bush envisaged this problem (see the quote starting this section).
[25] For example, navigating was a preferred method when searching for files but rarely used for bookmarks (O. Bergman et al., 2021) and relied upon but not effective for emails (Jones et al., 2014). With the advancement of indexing technologies there is overall tendency towards search-based method of refinding.
[26] Recently Microsoft enabled combined search in the files system, cloud storage and the web but, of course, at the price of using their browser.
[27] Some browsers, while they still have a separate search interface for bookmarks, include bookmarks in the search suggestions.
[28] From less than a dozen before 2020, in 2022 there were over 70 such tools. Some of them will not survive the next couple of years and the “birthrate” will most likely go down.
[29] Previously I referred to second wave as “PKG Apps” and I still find an appropriate alternative label.
[30] There are also tables for users, sharing documents, sharing docs and caching, but in terms of user-created content, there are only two.
[31] Recently discontinued.
[32] It is called “attribute” in Datalog/Datascript, yet it works more like an egde in a directed graph, than like an attribute in RDBMS terms.
[33] See https://github.com/kvistgaard/roamo
[34] See https://oasis-lab.gitbook.io/roamresearch-discourse-graph-extension/
[35] Logseq, a tool from the second group is also Markdown-based.
[36]Cmap is an exception and rather closer to the center. It even had a OWL-aware version, discontinued.
[37] See chapter 3 of Essential Balances (Velitchkov, 2020)
[38] For example, an issue can appear in the context where it was captured, a meeting, in the issues log and kanban board, in a presentation, and in an architecture diagram (Velitchkov, 2021b).
[39] Exaptation is a shift in a function of a trait during evolution. A classic example is bird feathers. Their initial function was temperature regulation. They then evolved for insect catching, sexual display, and finally for flight. Similar examples can be seen not only in the evolution of species but also in the evolution of technology. Maybe the most popular one is the discovery of the microwave oven. In 1945, a chocolate bar in the pocket of Percy Spencer at Raytheon started to melt when it was exposed to the microwaves from an active radar set.
[40] In my projects I started treating GitHub and GitLab as graphs, stimulated by the use of GraphQL. This is also an example of exaptation since I’m using issues for various project items such as risks, principles, rules, decisions, and so on, through labelling and workflow conventions.
[41] See Twemex (https://tweethunter.io/) and Thread Portal for Twitter, and Side Portal for Wikipedia.
[42] The widespread adoption of radio a television in the middle of the last century had a similar effect. It led to passive consumption of information:
The viewer of television, the listener to radio, the reader of magazines, is presented with a whole complex of elements – all the way from ingenious rhetoric to carefully selected data and statistics – to make it easy for him to “make up his own mind” with the minimum of difficulty and effort. But the packaging is often done so effectively that the viewer, listener, or reader does not make up his own mind at all. Instead, he inserts a packaged opinion into his mind, somewhat like inserting a cassette into a cassette player. He then pushes a button and “plays back” the opinion whenever it seems appropriate to do so. He has performed acceptably without having had to think. It is puzzling, however, to observe similar passivity, wide-spread lack of critical thinking in situations where the information consumers are also the main information providers.
(Adler & Doren, 1972)
[43] This centralization has several manifestations. In the web, it’s a new form of “monopoly on demand to commodify supply” (Brander, 2021) and overall a new form of capitalism (Zuboff, 2019). In parallel, there is centralization of infrastructure services, the cloud platforms, and centralization of corporate application services, leading to increased vendor lock-in and higher vulnerability due to the “single”-point-of-failure design.
[44] 11km (7 miles) is the distance between the Facebook and Google headquarters.
[45] An example is DNS, historically, but it also has decentralized alternatives, such as the named data networking, claiming substantial advantages.
[46] https://getdweb.net/
[47] See https://www.go-fair.org/fair-principles/
[48] Decoupling is an evolutionary trend in knowledge management. During the Renaissance scholars looking for better methods for knowledge organization realized that the representation of the world does not need to mirror the world and the order of knowledge does not need to mirror the knowledge (Cevolini, 2018). After knowledge was decoupled from the would and the index was decoupled from knowledge, the next decoupling innovation bringing open-ended exploration was the replacement of bound commonplace books with free index cards. In more recent years semantic knowledge graphs are again a matter of decoupling structure and semantics that are tightly coupled in traditional relational database systems (the so-called “schema-on-write”).
[49] See https://github.com/logseq/rdf-export
[50] In a survey by Stack Overflow in 2021, VSCode ranked as number one, selected by 71% of the over 82K participants in the survey, significantly ahead of all the rest (Stack Overflow Developer Survey 2021, 2021).
[51] https://github.com/blockprotocol/blockprotocol
[52] See Brander (2022) and https://github.com/subconsciousnetwork/noosphere
AI
[SYS]: You are a helpful assistant. Please write in Korean language.
[ME]: Shaun Gallagher 의 저작들 알려줘
[AI]: Sean Gallagher는 의식과 신체 경험, 인지 과학 분야에서 잘 알려진 철학자이자 학자입니다. 그의 저작 중 일부는 다음과 같습니다:
- “How the Body Shapes the Mind” (2005) - 이 책은 신체가 인간의 인지 및 의식에 어떻게 영향을 미치는지를 탐구합니다.
- “The Phenomenological Mind” (공저: Dan Zahavi, 2008) - 이 책은 현상학과 인지과학의 교차점을 탐구하며, 둘 사이의 상호작용을 설명합니다.
- “Enactivist Interventions: Rethinking the Mind” (2017) - Gallagher는 이 책에서 인지의 작동 방식을 다시 생각하고, 환경과의 적극적인 상호작용을 강조하는 참여적 관점을 제안합니다.
- “Action and Interaction” (2020) - 이 책은 사회적 상호작용이 인지와 행동에 미치는 영향을 탐구합니다.
Gallagher의 연구는 인지 과학, 현상학, 철학적 심리학 등 다양한 분야에 걸쳐 있으며, 그의 저작들은 이들 분야에 큰 기여를 하고 있습니다.
[ME]: